Latest Writings
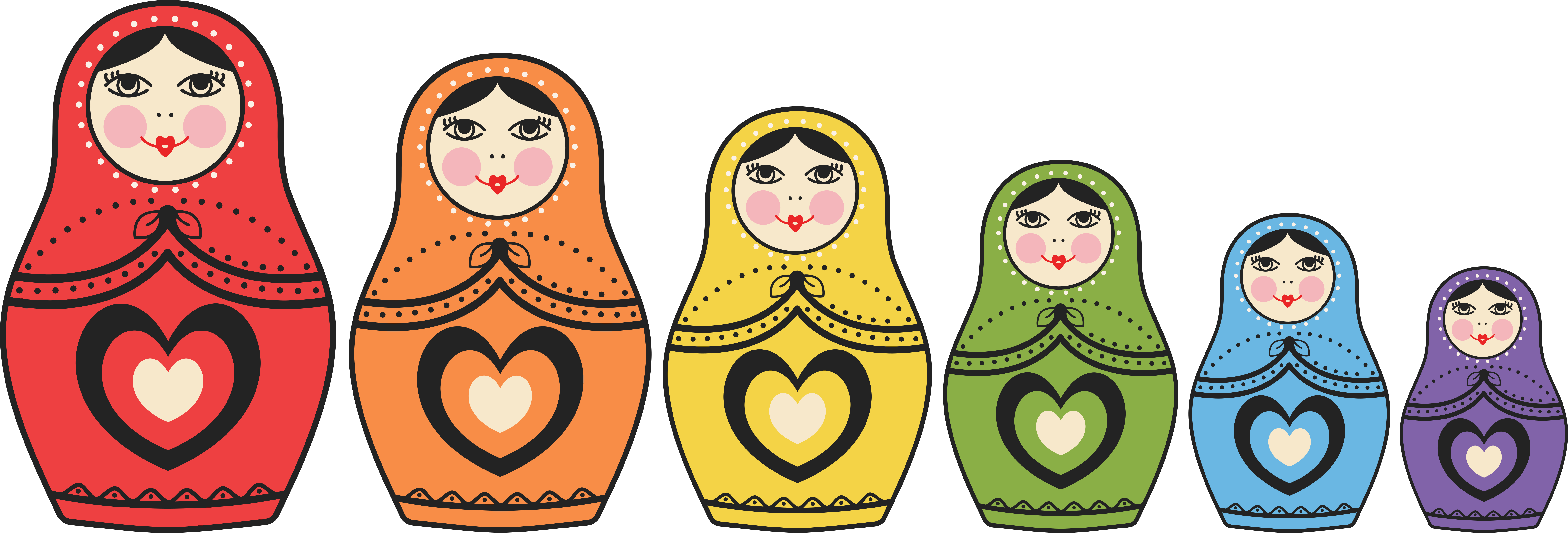
Matryoshka Representation Learning (MRL) is a flexible representation technique to learn representations by enforcing a course-to-fine structure over the embeddings by encoding information at multiple levels of granularity. Each granularity level comprises the first $m$ feature components of $z$. After training, users can choose different granularities of a representation based on the best use case while trading off the minimum accuracy possible.
Feb 23, 2024
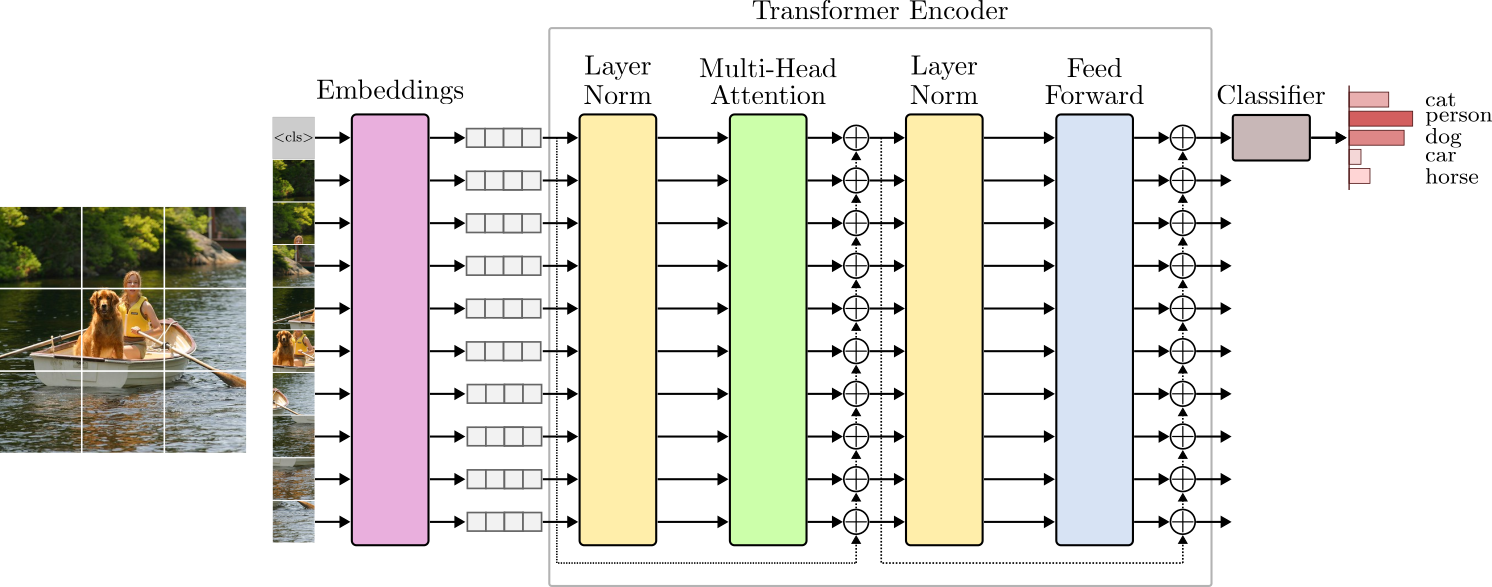
Since its proposal, the Transformer has taken deep learning by storm. Initially designed for solving sequence-to-sequence tasks such as machine translation, the Transformer is now everywhere, from NLP to audio, videos, and images. The Transformer unifies all perception tasks into a single architecture. The Transformer combines ideas from various research into an elegant design that has stood the test of time. Let's dive into its architecture and implement it in PyTorch.
Mar 15, 2023
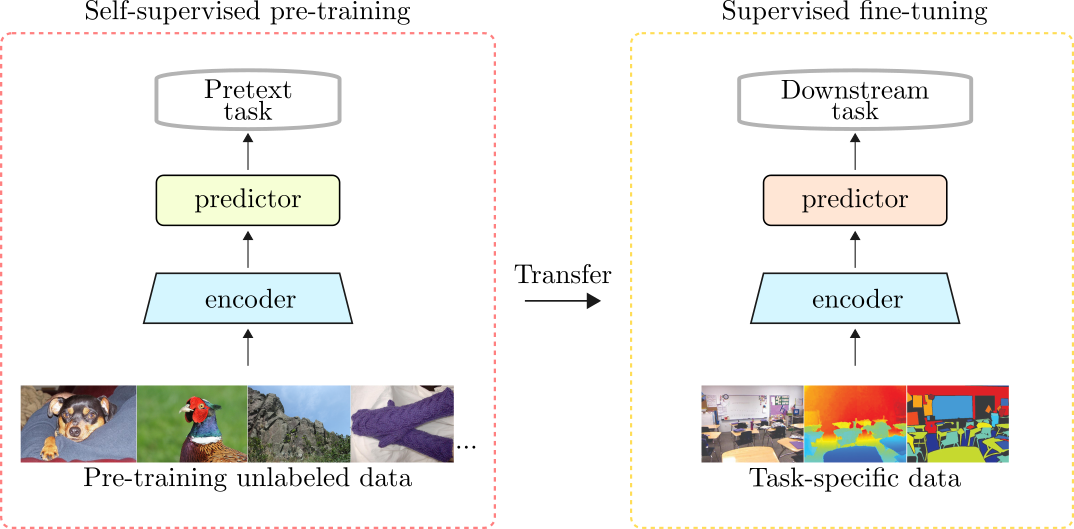
Representation learning aims to map a high-dimensional complex data point to a compact and low-dimensional representation. This low-level representation must be generalizable, i.e., it must ease the process of learning new downstream tasks to the extent that new tasks should require fewer labeled examples than it would if learning the same task from scratch.
Apr 5, 2022
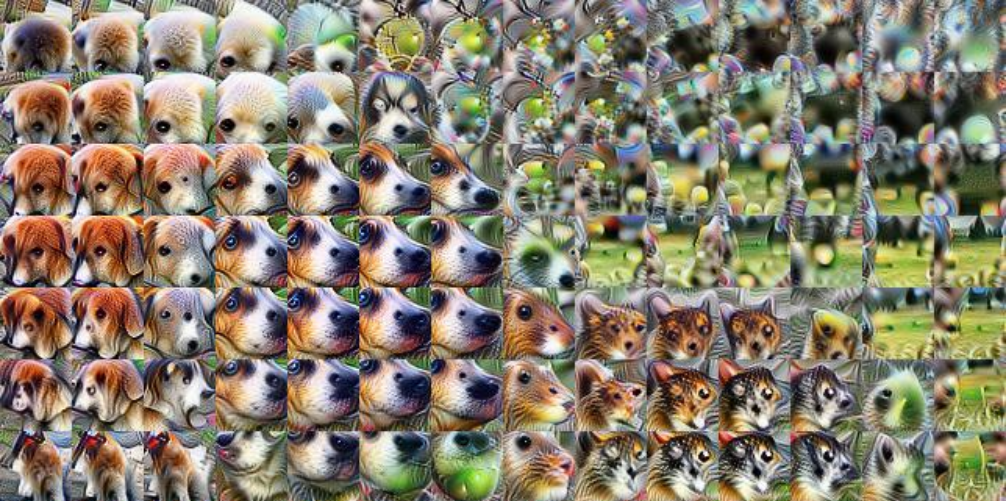
Representation learning aims to map high-dimensional data to often dense, compact, and generalizable representations. These learned representations can transfer well to other tasks and have been the principal method to solve problems in which data annotations are hard or even impossible to get.
Apr 15, 2021
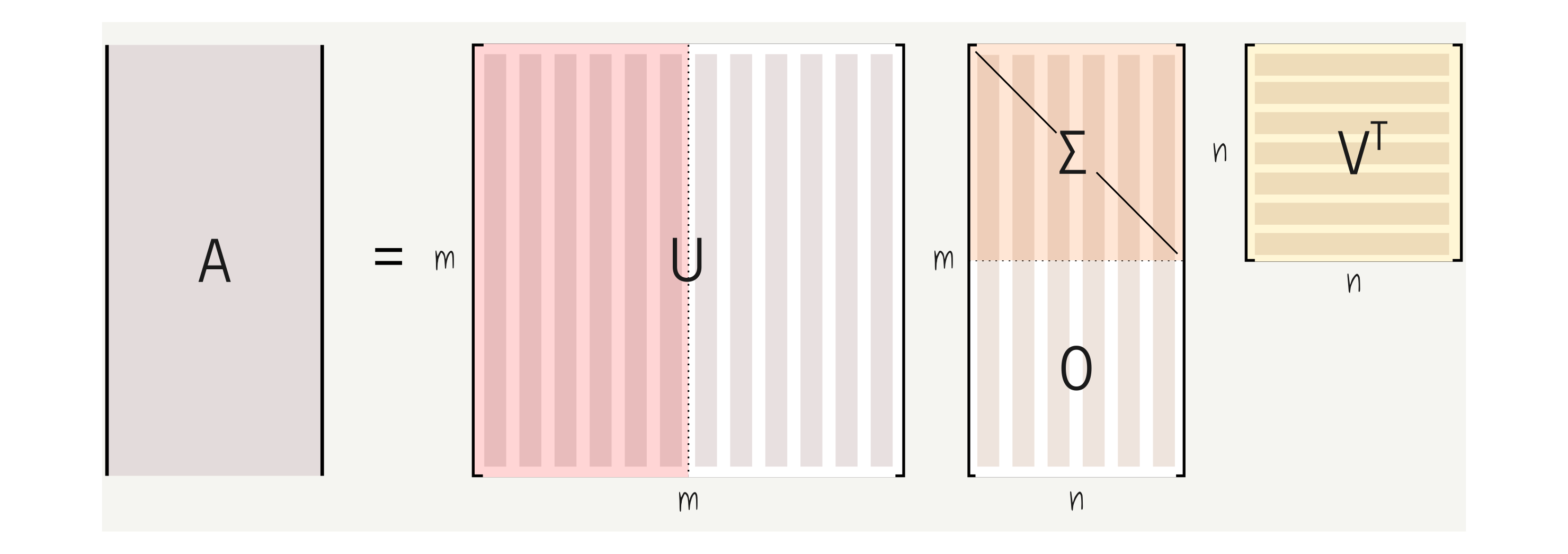
It is common to see educational material explaining linear regression using gradient descent. In this post, we will take on linear regression through the lens of linear algebra and solve it using the singular value decomposition (SVD).
Oct 12, 2020
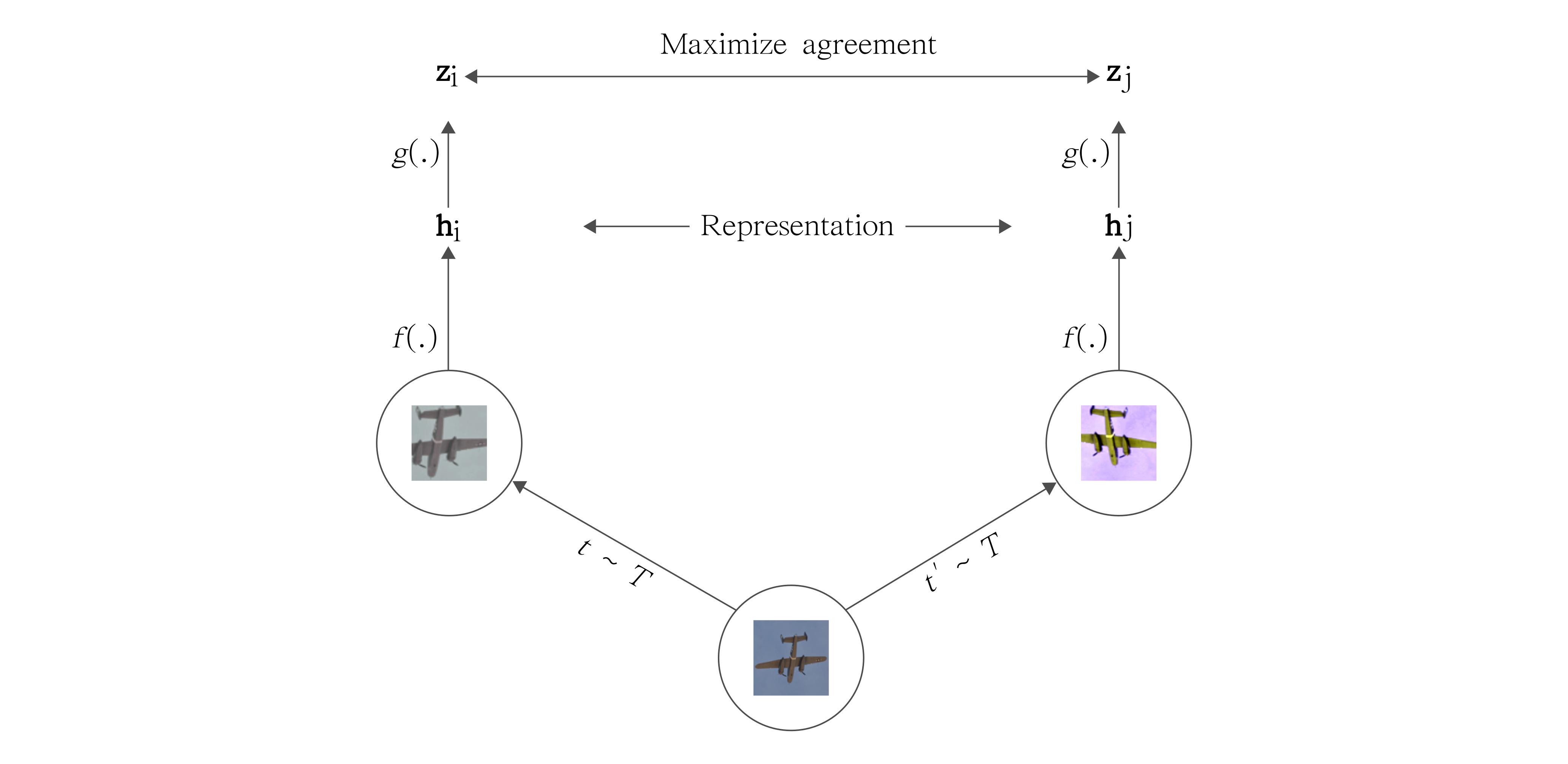
A word on contrastive learning and the recent success of unsupervised representation learning. We also build and explore SimCLR: A simple framework for contrastive learning.
Feb 23, 2020
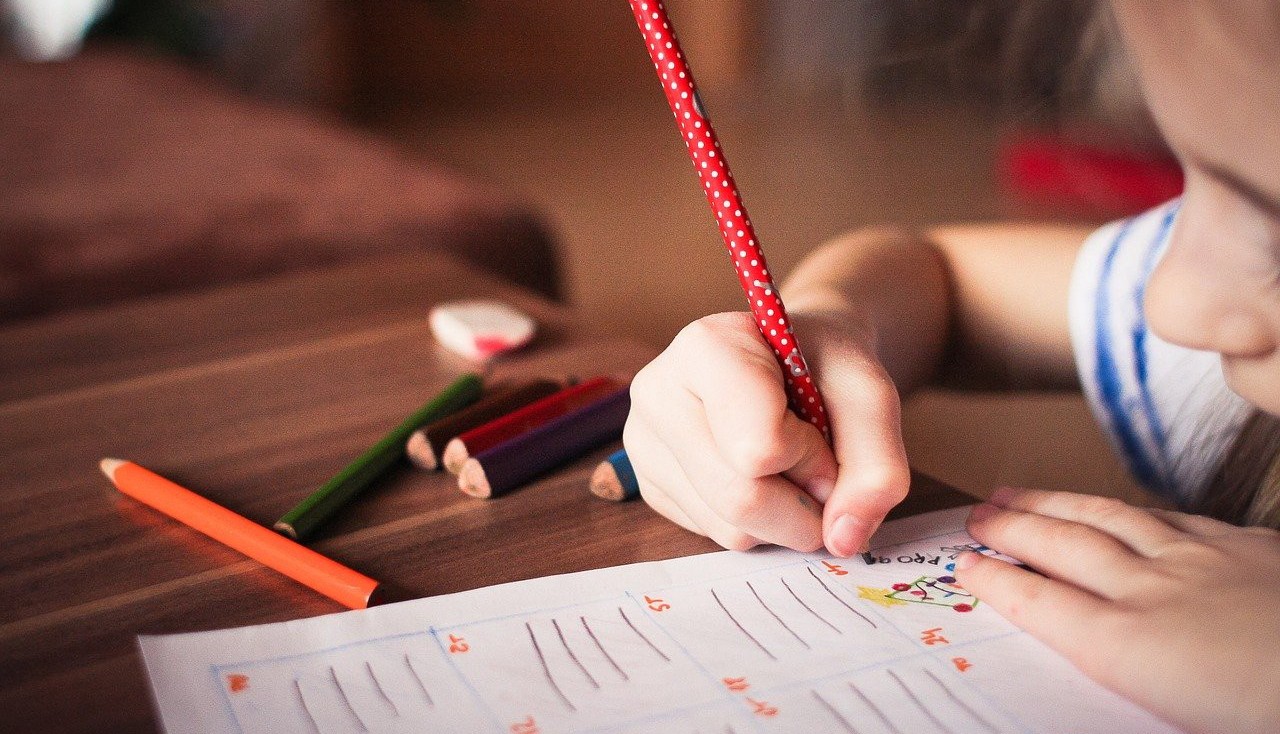
Notes on the current state of deep learning and how self-supervision may be the answer to more robust models
Jan 20, 2020
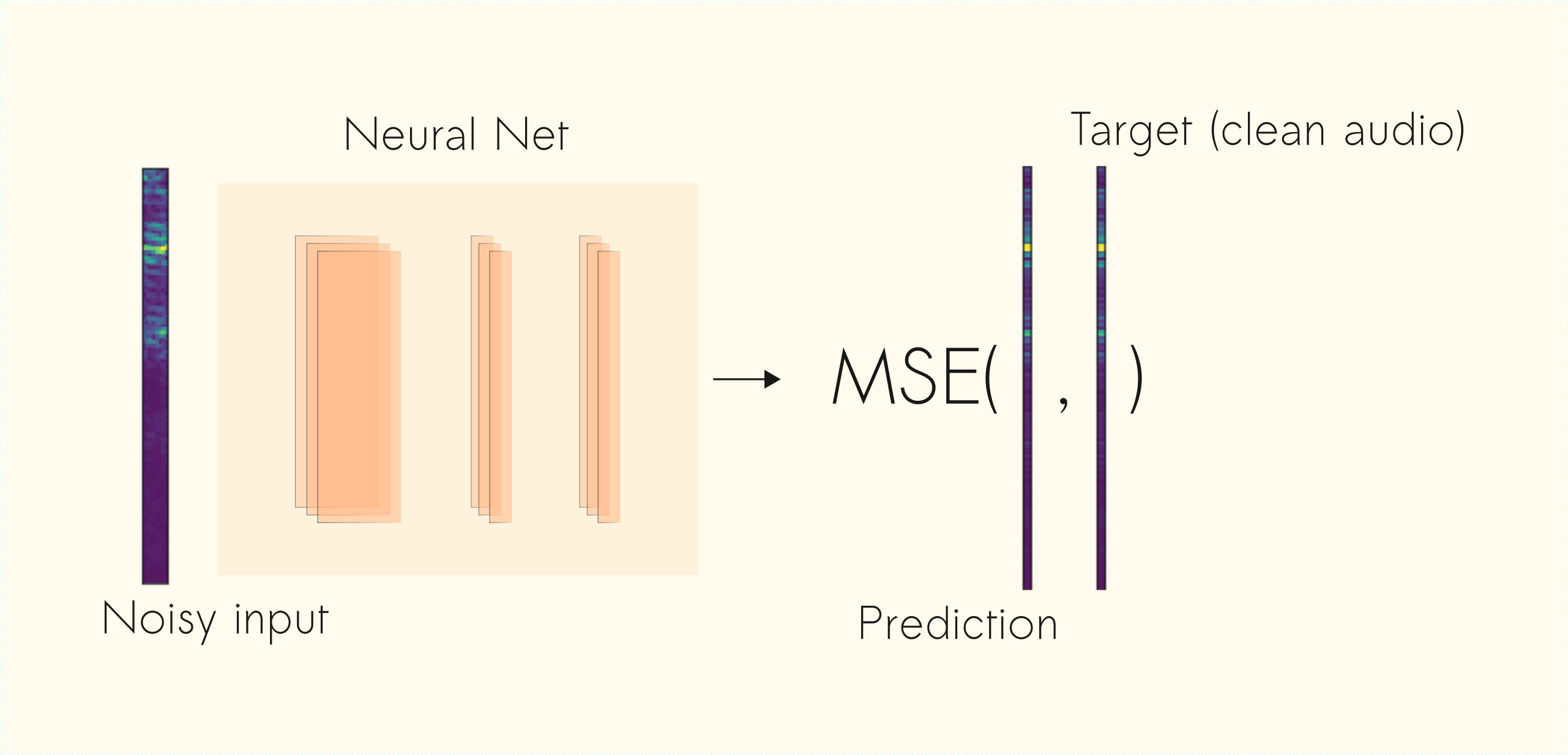
Speech denoising is a long-standing problem. In this article, we use Convolutional Neural Networks (CNNs) to tackle this problem. Given a noisy input signal, we aim to build a statistical model that can extract the clean signal (the source) and return it to the user.
Dec 18, 2019
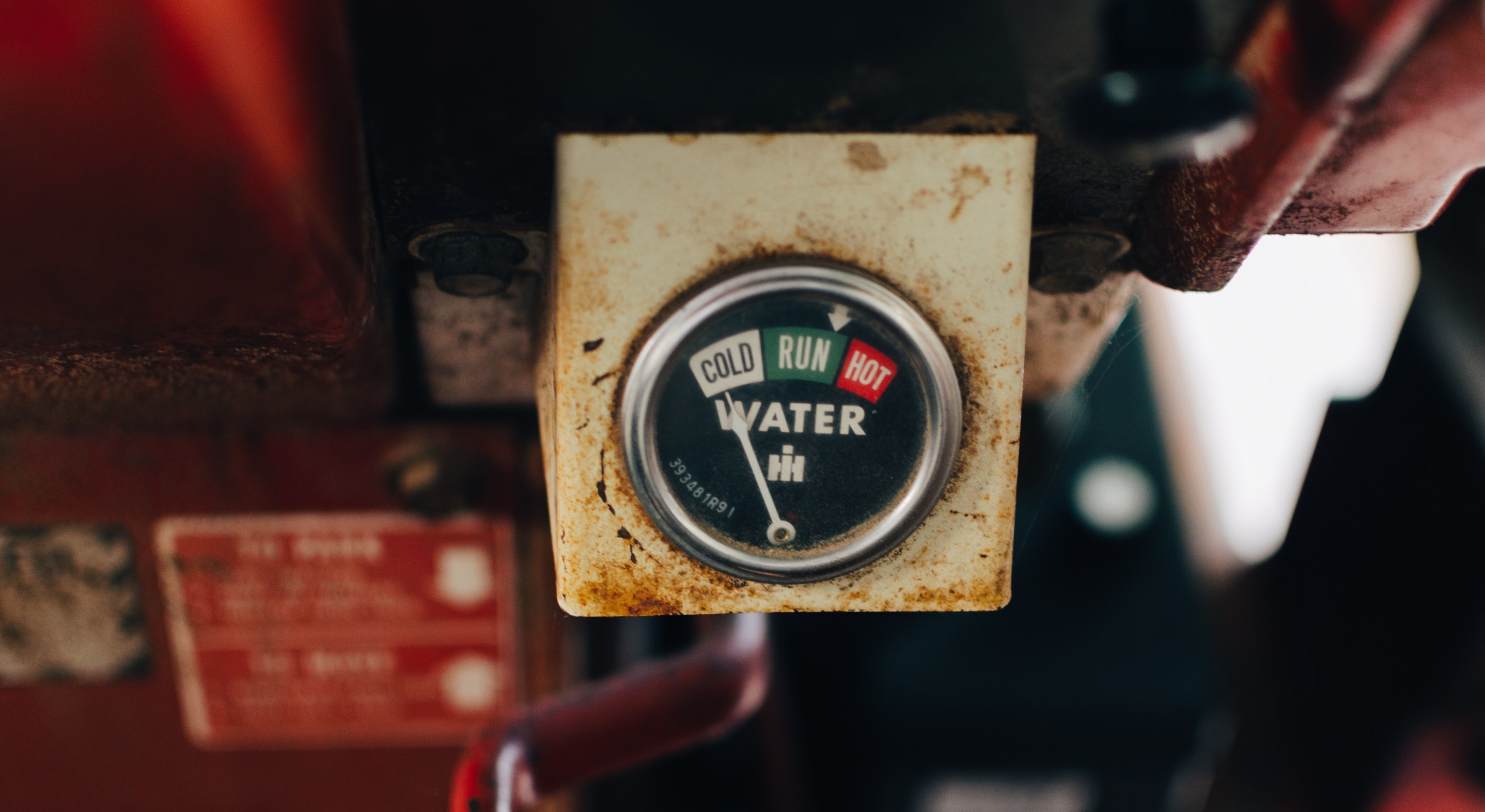
If you train deep learning models for a living, you might be tired of knowing one specific and important thing: fine-tuning deep pre-trained models requires a lot of regularization.
Nov 26, 2019
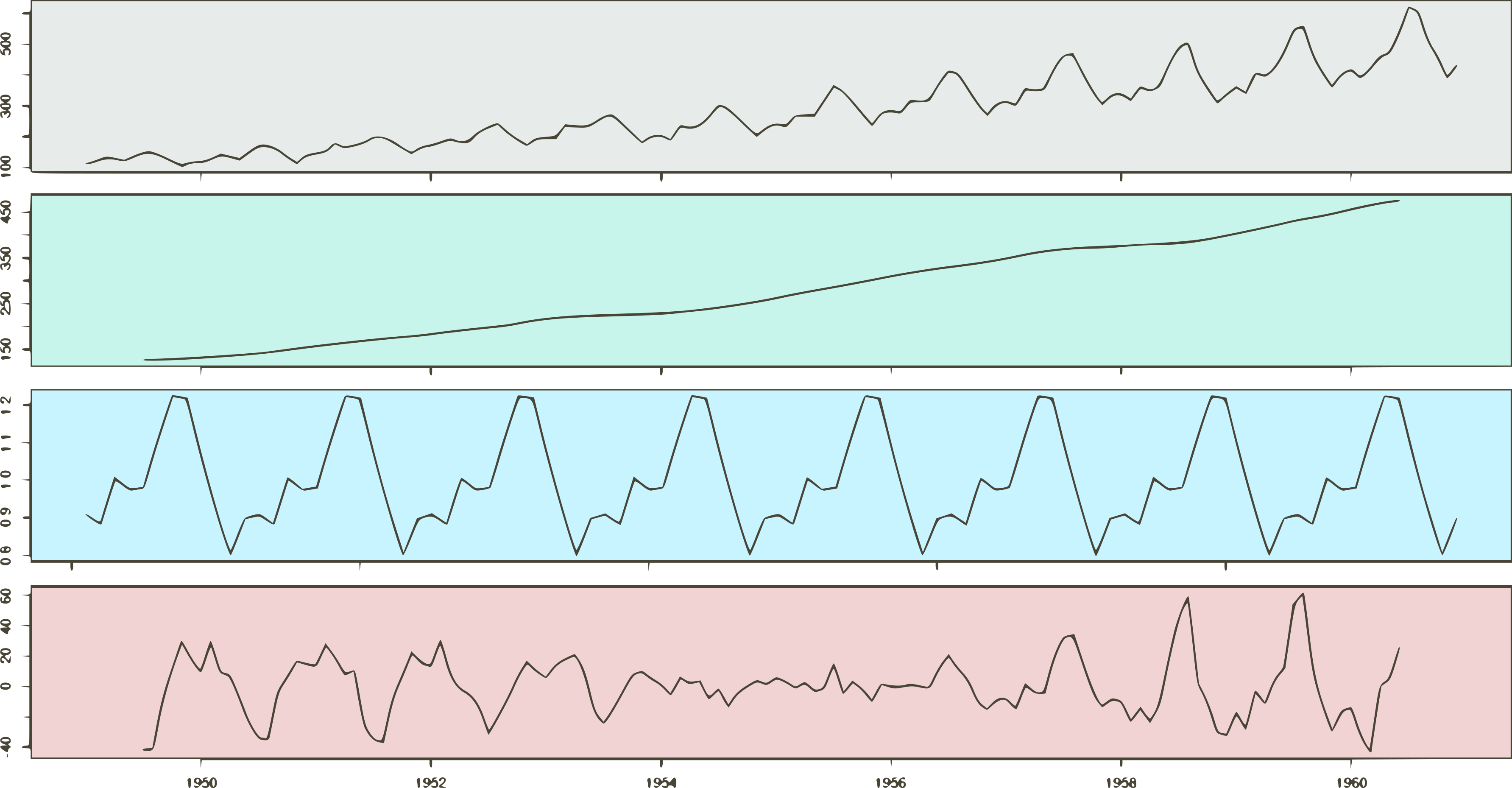
Time Series Forecasting is the use of statistical methods to predict future behavior based on a series of past data. Simply put, we can think of it as a bunch of values collected through time. In this post, we explore two decomposition methods: additive and multiplicative decomposition.
Aug 8, 2019
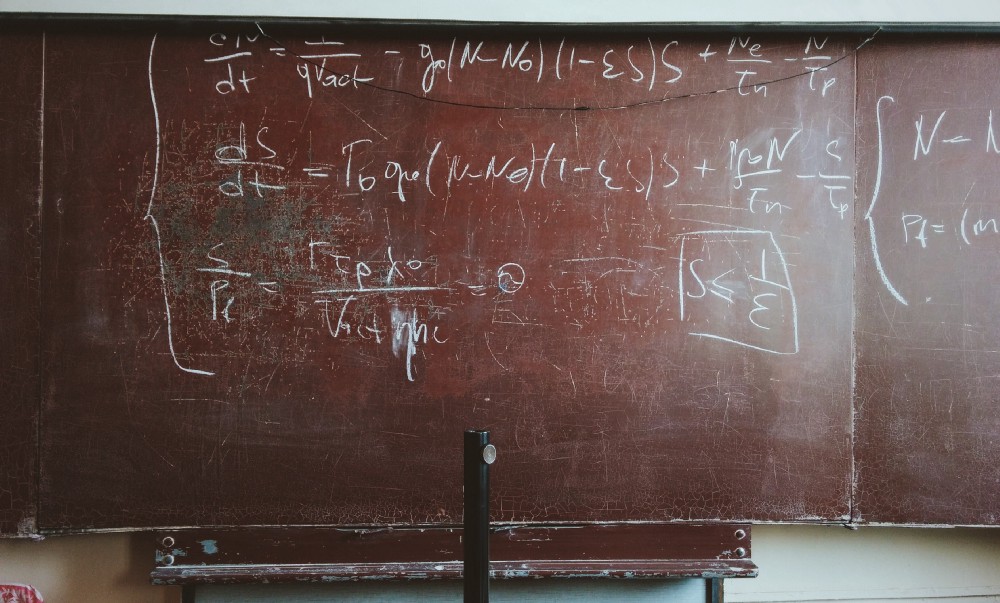
We can categorize ML models based on the way they classify data. There are two types: generative and discriminative methods. Let's dive deeper into one of the most popular discriminative models - Logistic Regression.
Feb 16, 2019
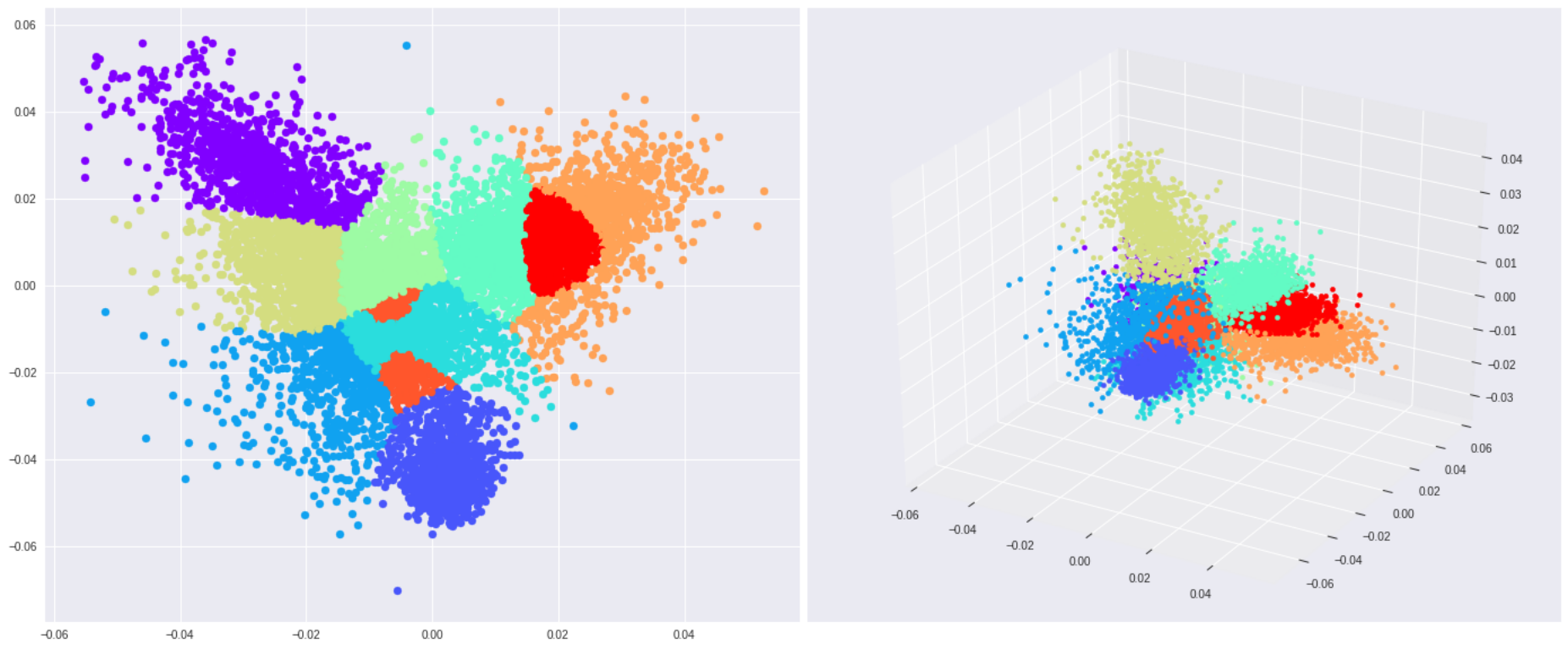
To deal with problems with 2 or more classes, most ML algorithms work the same way. Usually, they apply some kind of transformation to the input data. The goal is to project the data to a new space. Then, they try to classify the data points by finding a linear separation.
Jan 3, 2019
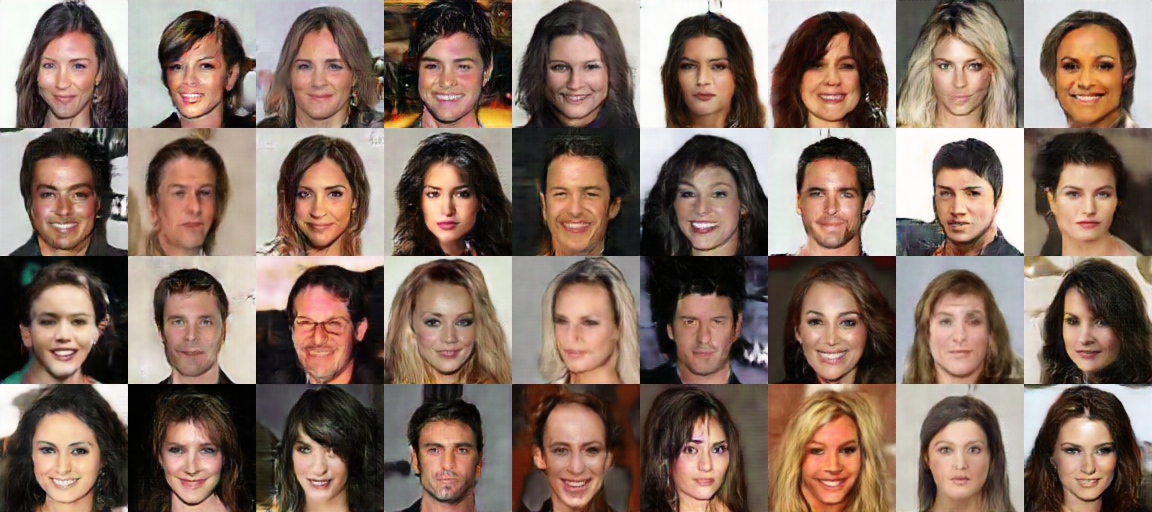
Lately, Generative Models are drawing a lot of attention. Much of that comes from Generative Adversarial Networks (GANs). Let's investigate some recent techniques for improving GAN training.
Aug 11, 2018
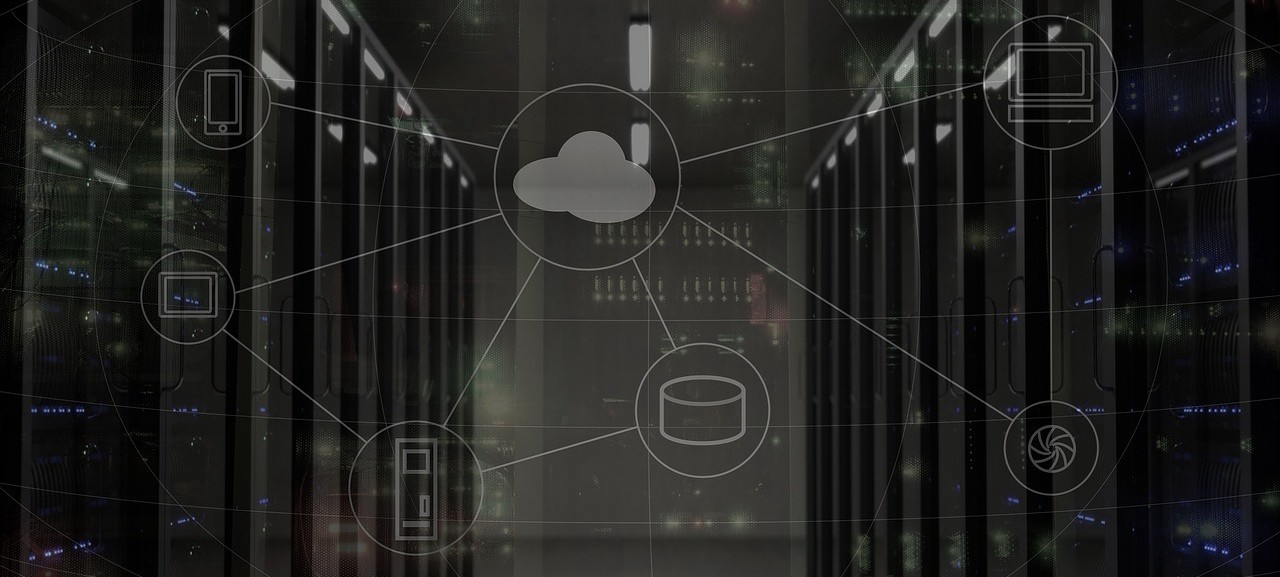
Putting Machine Learning (ML) models to production has been a recurrent topic. To address this concern, TensorFlow (TF) Serving is Google’s best bet for deploying ML models to production.
Jun 18, 2018
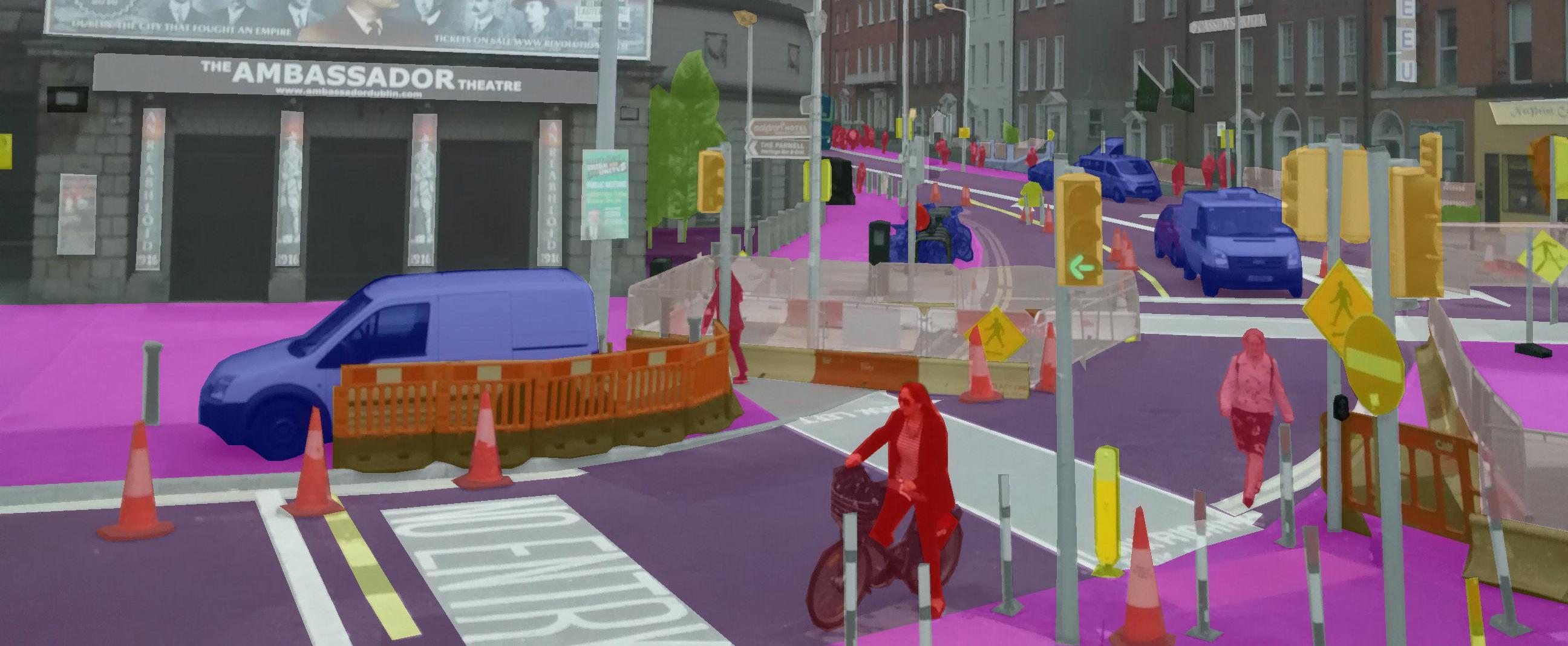
Deep Convolution Neural Networks (DCNNs) have achieved remarkable success in various Computer Vision applications. Like others, the task of semantic segmentation is not an exception to this trend.
Jan 29, 2018
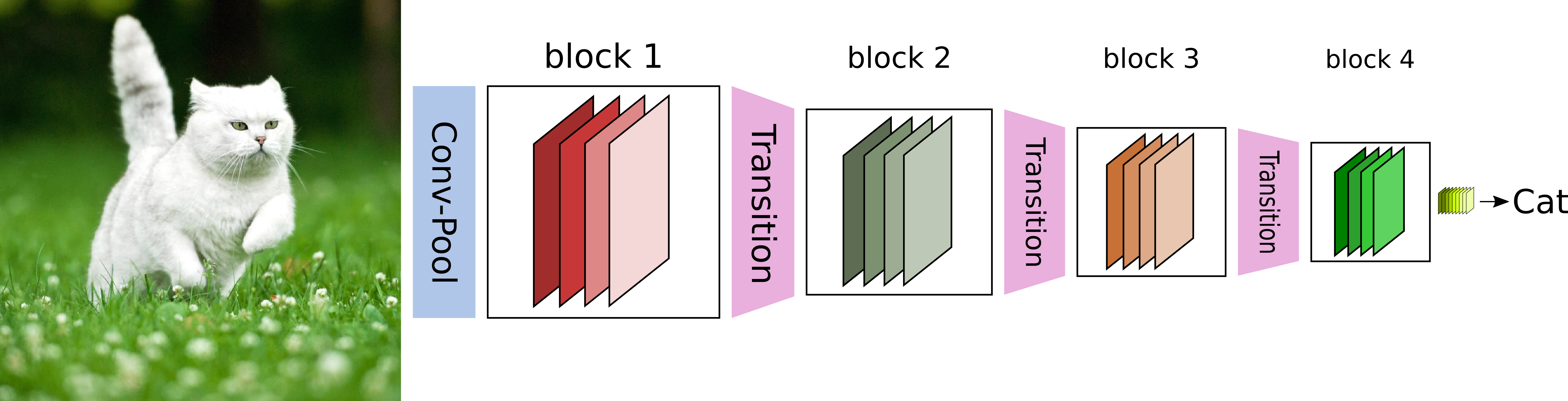
DenseNets offer very scalable models that achieve very good accuracy and are easy to train. The key idea consists sharing feature maps within a block through direct connections between layers.
Dec 12, 2017
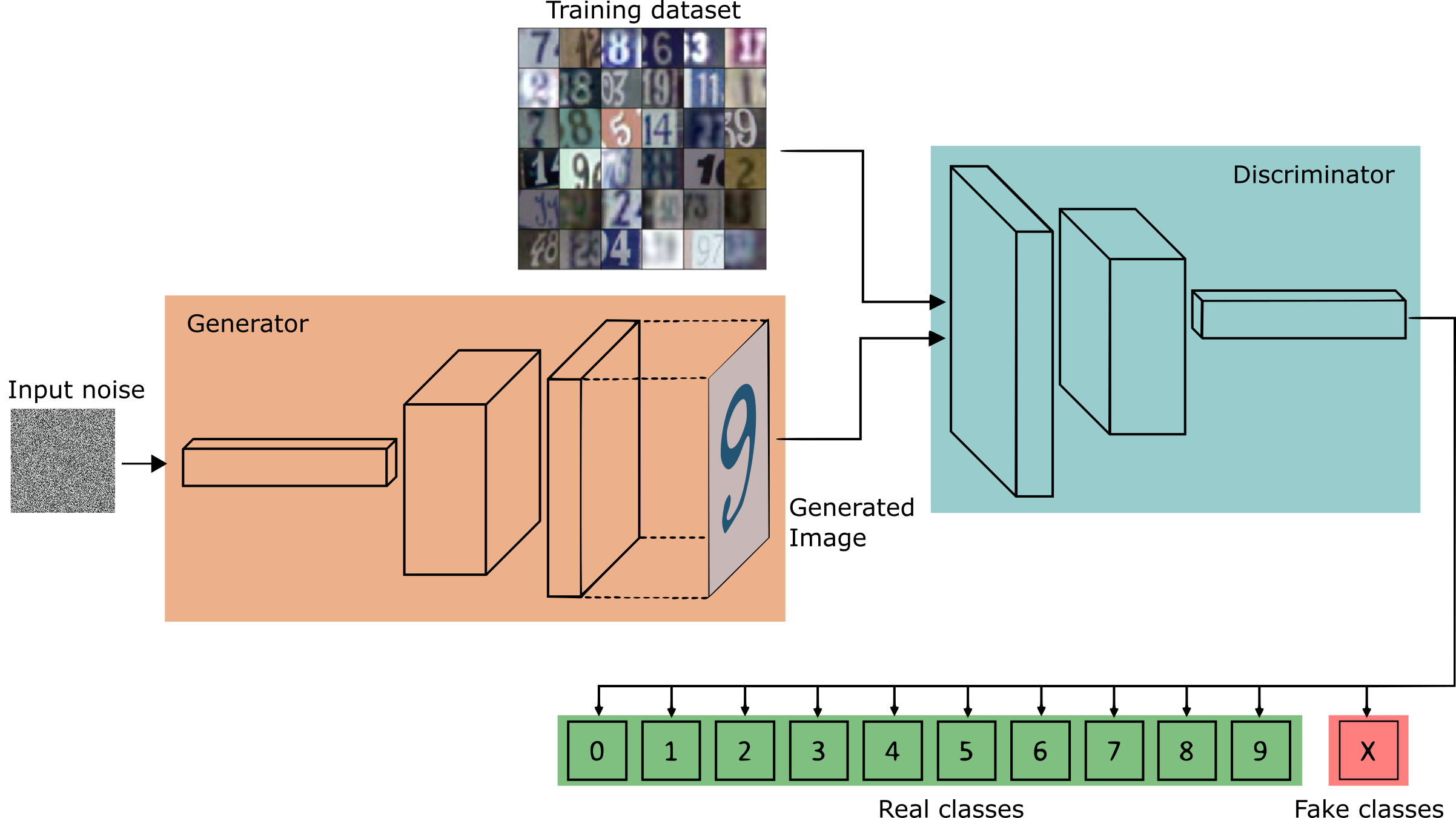
Supervised learning has been the center of most researching in deep learning in recent years. However, the necessity of creating models capable of learning from fewer or no labeled data is greater year by year.
Jul 31, 2017
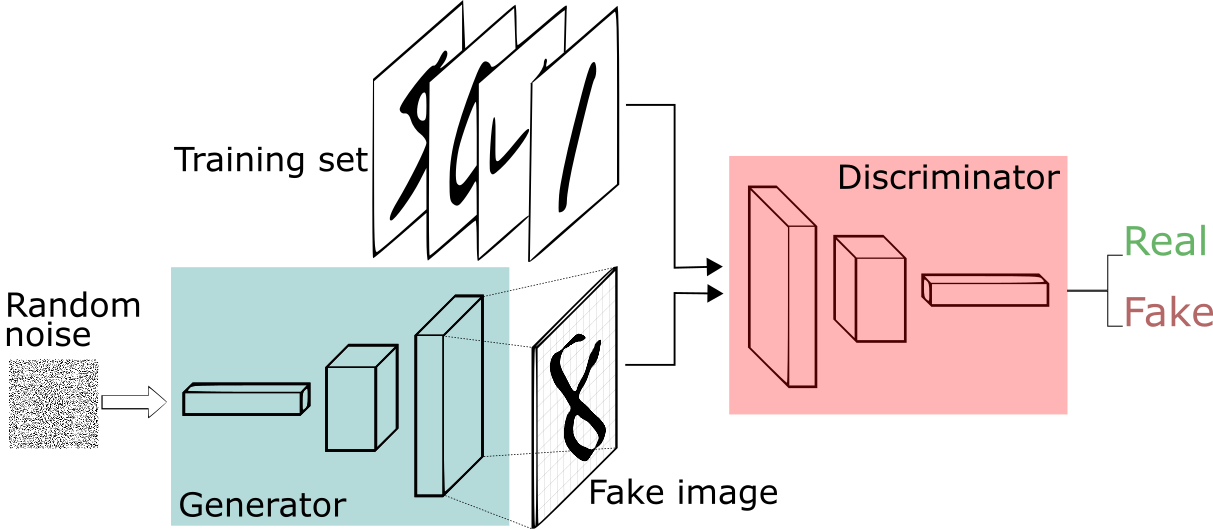
GANs are a kind of generative model in which two differentiable functions are locked in a game. The generator tries to reproduce data that come from some probability distribution. The discriminator gets to decide if its input comes from the generator or from the true training set.
Jun 7, 2017